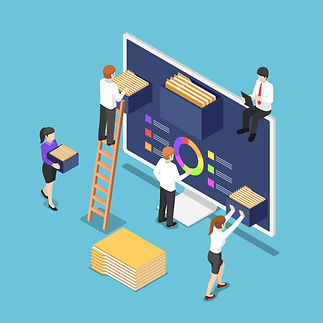
Data Management
Data management is a crucial discipline in the digital age, encompassing the processes, practices, and tools used to collect, store, organize, and maintain data effectively. It ensures that data is accurate, accessible, and secure, enabling organizations to leverage it for decision-making, strategic planning, and operational efficiency. Key aspects of data management include data governance, which establishes policies and standards for data usage; data quality management, which focuses on maintaining high data accuracy and consistency; and data integration, which involves combining data from different sources to provide a unified view. Effective data management is essential for deriving meaningful insights, driving innovation, and maintaining a competitive edge in today's data-driven world.
Data Governance
Policies and Procedures
-
Establish Clear Guidelines: Develop comprehensive policies that outline how data should be handled, stored, accessed, and shared within the organization. These guidelines ensure consistent data practices and help mitigate risks.
-
Security Protocols: Define protocols to safeguard data against unauthorized access, breaches, and other security threats. This includes encryption standards, access controls, and incident response procedures.
Roles and Responsibilities
-
Data Stewards: Assign individuals or teams responsible for overseeing data quality, integrity, and lifecycle management. Data stewards ensure compliance with data governance policies and act as custodians of data within their domains.
-
Data Governance Officers: Appoint dedicated officers to oversee the overall governance framework, ensuring alignment with organizational objectives and regulatory requirements.
Compliance and Privacy
-
Regulatory Compliance: Implement measures to comply with relevant data protection regulations, such as GDPR (General Data Protection Regulation) and CCPA (California Consumer Privacy Act). This includes data handling practices, consent management, and data subject rights.
-
Data Audits: Regularly conduct audits to assess compliance with data governance policies and identify areas for improvement. Audits help ensure accountability and transparency in data management.
Data Standards
-
Consistency and Uniformity: Develop and enforce data standards to ensure consistency in data definitions, formats, and naming conventions across the organization. Standardization reduces ambiguity and enhances data quality.
-
Documentation: Maintain comprehensive documentation of data standards and procedures to facilitate understanding and adherence by all stakeholders.
Data Quality Management
Data Accuracy
-
Validation Processes: Implement rigorous data validation processes to check for errors, inconsistencies, and inaccuracies. This may include automated checks, manual reviews, and data cleansing tools.
-
Correction Mechanisms: Establish mechanisms for detecting and correcting errors promptly. This includes automated error correction tools and manual intervention when necessary.
Data Completeness
-
Identify Gaps: Use data profiling techniques to identify missing data elements and assess the completeness of datasets.
-
Data Enrichment: Enhance data completeness by integrating additional data from external sources or through data enrichment services, filling in gaps and providing more comprehensive information.
Data Reliability
-
Dependability: Ensure that data is consistently available and reliable for decision-making. This involves maintaining robust data infrastructure and minimizing downtime.
-
Source Monitoring: Continuously monitor data sources and systems to ensure they are functioning correctly and providing accurate data.
Data Timeliness
-
Up-to-Date Information: Implement processes to ensure data is updated regularly and reflects the latest information. This is crucial for real-time decision-making and analytics.
-
Real-Time Processing: Where applicable, utilize real-time data processing and updating mechanisms to provide timely and relevant data.
Continuous Monitoring
-
Automated Checks: Set up automated data quality checks and alerts to continuously monitor data quality. These systems can detect anomalies and trigger corrective actions.
-
Regular Reviews: Conduct regular reviews and updates of data quality metrics and standards to ensure they remain relevant and effective.
Data Integration and Connectivity
Data Integration
-
Unified View: Combine data from multiple sources to create a unified, comprehensive view that supports better analysis and decision-making.
-
ETL Processes: Use ETL (Extract, Transform, Load) processes to extract data from various sources, transform it into a consistent format, and load it into a central repository. This ensures data is harmonized and ready for use.
-
Data Consistency: Ensure that integrated data maintains consistency and integrity across different systems and sources.
Connectivity
-
System Communication: Enable different systems and applications to communicate and exchange data seamlessly. This requires adopting standard protocols and data formats.
-
Standard Protocols: Use APIs (Application Programming Interfaces), XML (eXtensible Markup Language), and JSON (JavaScript Object Notation) to facilitate interoperability and data exchange.
Centralized Data Management
-
Data Warehouse/Lake: Implement a centralized data warehouse or data lake to store and manage integrated data. This provides a single source of truth and simplifies data access.
-
Seamless Access: Ensure that data is easily accessible to authorized users across the organization, supporting data-driven decision-making and analysis.
Data Harmonization
-
Standardization: Standardize data from diverse sources to ensure uniformity and coherence. This involves resolving conflicts and discrepancies in data definitions and formats.
-
Conflict Resolution: Implement processes to identify and resolve conflicts in data from different origins, ensuring a consistent and reliable dataset.
Scalability
-
Handling Growth: Design data integration solutions that can scale with increasing data volumes and complexity. This involves optimizing performance and ensuring the infrastructure can handle large datasets efficiently.
-
Performance Optimization: Continuously optimize data integration processes to maintain performance and efficiency as data volumes grow.